Article By : CEA-Leti
Impressed by the barn owl’s neuroanatomy, CEA-Leti has developed an event-driven, object-localization system that {couples} state-of-the-art piezoelectric, ultrasound transducer sensors…
Impressed by the barn owl’s neuroanatomy, CEA-Leti, the know-how analysis institute primarily based in Grenoble (France), has developed an event-driven, object-localization system that {couples} state-of-the-art piezoelectric, ultrasound transducer sensors to a neuromorphic, resistive memories-based computational map.
Introduced in a paper revealed lately in Nature Communications, the analysis group describes growth of an auditory-processing system that will increase power effectivity by as much as 5 orders of magnitude in comparison with standard localization techniques.
“Actual-world sensory-processing functions require compact, low-latency, and low-power computing techniques,” the paper, “Neuromorphic Object Localization Utilizing Resistive Recollections and Ultrasonic Transducers”, explains. “Enabled by their in-memory, event-driven computing skills, hybrid memristive-complementary metal-oxide semiconductor (CMOS) neuromorphic architectures present a great {hardware} substrate for such duties.”
[Download] RF Sign Chain Discourse: Properties and Efficiency Metrics
Neurobiology presents a spectrum of ultralow-power options to effectively course of sensory info, as completely different animals and bugs have developed to successfully carry out troublesome duties with restricted energy. On the coronary heart of organic sign processing are two elementary ideas: event-driven sensing and analog in-memory computing.
“We drew inspiration from biology to include these two features of computation into our {hardware}, leveraging CEA-Leti’s state-of-the-art ultrasound sensors and resistive reminiscence applied sciences,” mentioned Elisa Vianello, senior scientist and Edge AI program coordinator, and senior creator of the paper. “Specifically, we targeted on the acoustic-based, object-localization activity. Owls effectively remedy this drawback and thus we extrapolated their computational ideas into our system.”
CEA-Leti constructed and examined this object localization system with the assistance of CEA-Checklist, College of Zurich, College of Excursions and College of Udine researchers. The group leveraged CEA-Leti’s successes in creating piezoelectric micromachined ultrasound transducer (pMUT) sensors and its developments in spiking neural networks primarily based on resistive reminiscence applied sciences.
The researchers’ first problem was creating a pre-processing pipeline that extracts the important thing info from pMUTs, which encode info primarily based on transient occasions or spikes. This temporal sign coding results in increased energy-efficiencies in comparison with conventional steady analogue or digital information, in order that solely related information are processed.
‘Bio-inspired analog RRAM-based circuit’
“Our system, which might have future use in sensor-fusion functions, mimics the owl’s extraordinarily energy-efficient prey-capture mechanism, which is preceded by mixed auditory and visible search,” mentioned Filippo Moro, lead creator of the paper. “The ultralow energy consumption auditory search is all the time energetic and when a selected auditory neuron fires, the owl has the knowledge it wants to begin the visible search, which is extra exact however extra expensive when it comes to power consumption.”
The second problem was designing and fabricating a bio-inspired analog RRAM-based circuit to effectively course of the extracted occasions and estimate an object’s location. Resistive reminiscence gives a compact answer to retailer the synaptic weights and RRAMs are non-volatile units, a function that matches the asynchronous event-driven nature of the group’s proposed system, leading to no energy consumption when the system is idle.
“To attenuate the power consumption of the thing localization system, researchers envisioned, designed, and carried out an environment friendly RRAM-based neuromorphic circuit that processes sign info produced by embedded sensors to calculate a focused object’s place in actual time,” the paper reviews. “Whereas standard processing strategies would constantly pattern the detected sign and crunch calculations to extract the helpful info, the proposed neuromorphic answer computes asynchronously because the helpful info arrives: this has allowed us to extend the system’s power effectivity by as much as 5 orders of magnitude.”
Over the previous decade, CEA-Leti has made substantial progress in pMUT sensors and spiking neural networks primarily based on resistive reminiscence applied sciences. The present work exhibits that combining visible sensors, equivalent to DVS cameras, and the proposed pMUT-based audition sensor must be explored to develop future shopper robotics.
As well as, mimicking a barn owl’s exact and environment friendly object-localization system is one other instance of the institute’s work to show that bio-inspired ideas can dramatically enhance efficiency of Edge-AI techniques. In March, Vianello acquired a €3 million grant from the European Analysis Council (ERC) to construct nanoscale reminiscence units impressed by insect nervous techniques for such functions as shopper robotics, implantable medical diagnostic microchips and wearable electronics.
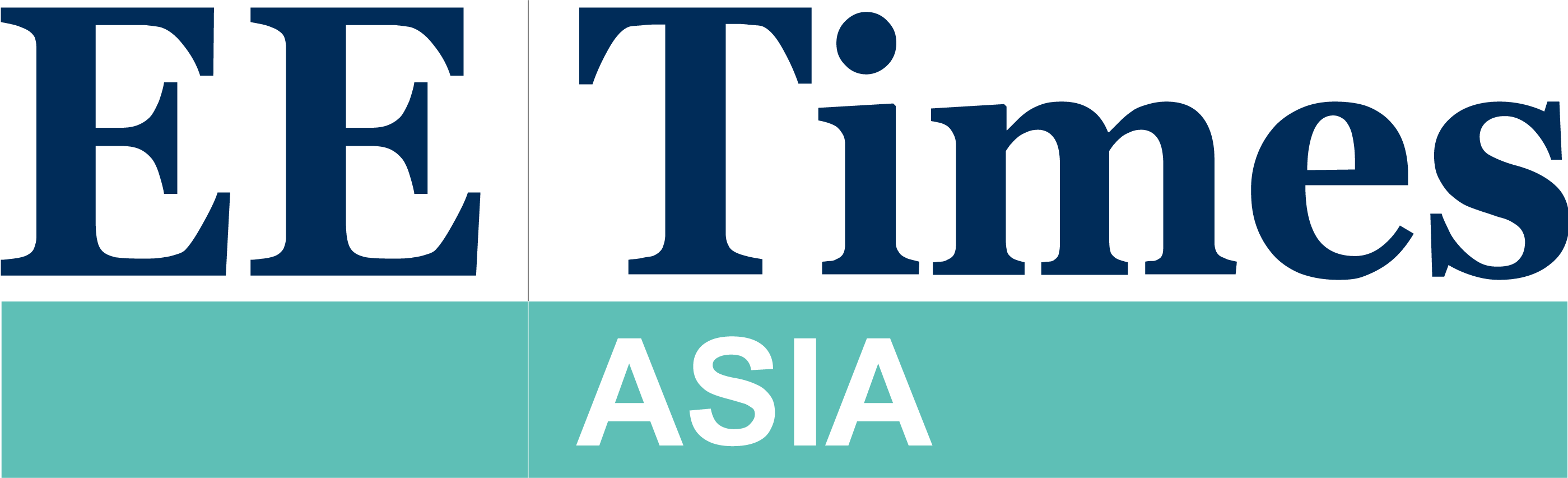